Deep Learning: The Revolution in Artificial Intelligence and Its Impact on Technology
- Connor Mcgovern
- Jun 7, 2023
- 5 min read
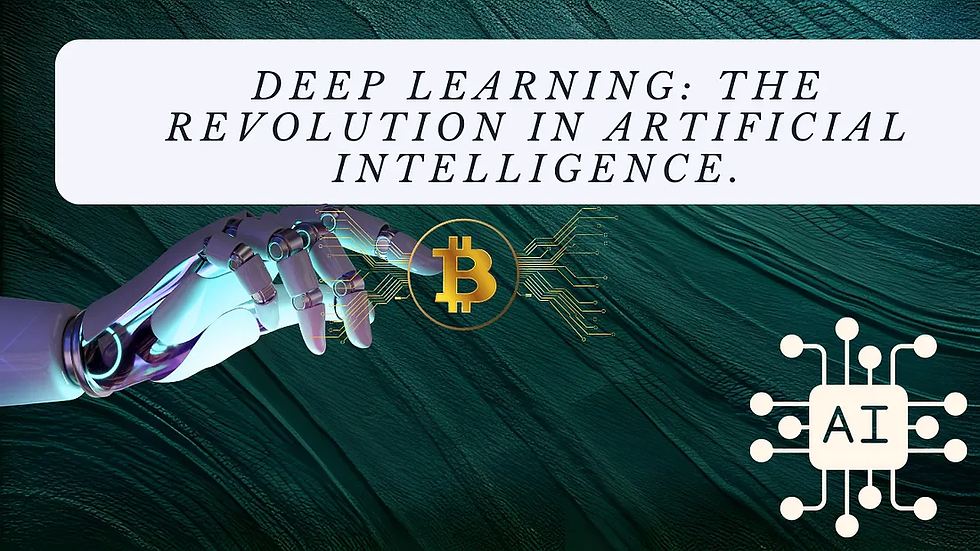
Deep Learning: The Revolution in Artificial Intelligence and Its Impact on Technology Welcome.
Deep learning is a machine learning technique that enables computers to learn from data and perform tasks that were once thought to be exclusive to humans. By leveraging large amounts of data and powerful computing resources, deep learning has significantly advanced the fields of computer vision, natural language processing, and more. As a result, deep learning is becoming increasingly central to the development and deployment of cutting-edge technologies, from driverless cars to voice-controlled devices.
The Emergence of Deep Learning Deep learning is a subset of machine learning, which itself is a part of the broader field of artificial intelligence (AI). AI has been a topic of fascination and research since the 1950s, with scientists and researchers aiming to create intelligent machines that can perform tasks without human intervention. However, significant progress in AI had been limited, especially in achieving the goal of “General AI,” intelligent machines that possess all human senses and reasoning capabilities. The introduction of deep learning techniques in recent years has revolutionized the field of AI, enabling the development of “Narrow AI” technologies, which can perform specific tasks as well or better than humans. Examples of Narrow AI include image classification, speech recognition, and natural language processing.
The Fundamentals of Deep Learning At its core, deep learning involves using artificial neural networks to model complex patterns and relationships in data. These networks consist of multiple layers of interconnected nodes, organized in a hierarchical structure. As data passes through the network, each layer processes the input and passes the result to the next layer, ultimately producing a final output. The primary advantage of deep learning over traditional machine learning techniques is its ability to automatically extract relevant features from raw data. This eliminates the need for manual feature extraction, which can be time-consuming and prone to errors. One popular type of deep neural network is the convolutional neural network (CNN), which is particularly suited to processing 2D data such as images. CNNs have been instrumental in advancing the field of computer vision, allowing for highly accurate object classification and recognition.
The Role of Data and Computing Power in Deep Learning Two key factors have contributed to the success of deep learning: the availability of large amounts of labeled data and the rise of high-performance computing resources, particularly graphics processing units (GPUs). The combination of these factors has enabled researchers to train deep learning models on vast datasets, achieving state-of-the-art accuracy and even surpassing human-level performance in some cases.
Large Datasets Deep learning models require substantial amounts of labeled data to achieve high levels of accuracy. For example, the development of driverless cars relies on millions of images and thousands of hours of video footage to train the underlying deep-learning models. Similarly, medical researchers are using deep learning to automatically detect cancer cells in high-dimensional microscopy data.
GPUs and Parallel Computing The computational demands of deep learning are immense, with training times often measured in days or even weeks. However, the parallel architecture of GPUs has proven to be highly efficient for deep learning tasks, enabling researchers to reduce training times significantly. The use of GPUs in combination with cloud computing resources has further accelerated the development of deep learning models, bringing the field to the forefront of AI research.
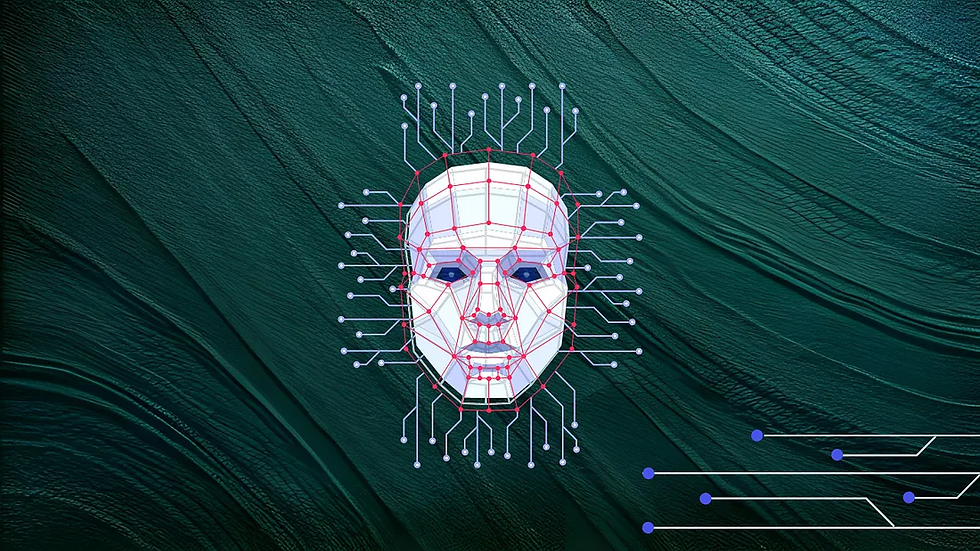
Applications of Deep Learning in Various Industries Deep learning is being used across a wide range of industries, transforming how we live and work. Some notable examples include:
Automated Driving Automotive researchers are using deep learning to detect objects such as stop signs and traffic lights, as well as to identify pedestrians and other obstacles. This technology is crucial for improving the safety and reliability of driverless vehicles.
Aerospace and Defense Deep learning is being used to analyze satellite imagery, identifying areas of interest and potential threats. This technology can help military and intelligence agencies make more informed decisions and improve overall situational awareness.
Medical Research Deep learning is being used to aid in the diagnosis and treatment of various medical conditions, including cancer. By automatically detecting cancer cells in medical imagery, deep learning models can help doctors make more accurate diagnoses and develop more effective treatment plans.
Industrial Automation Deep learning is being employed to improve worker safety around heavy machinery by automatically detecting when people or objects are within an unsafe distance. This technology can help prevent accidents and improve overall workplace safety.
Electronics and Voice Control Deep learning is powering the development of voice-controlled devices, such as smart speakers and virtual assistants. By accurately recognizing and interpreting spoken commands, these devices can provide users with a seamless and intuitive user experience.
Machine Learning vs. Deep Learning: Key Differences While deep learning is a specialized form of machine learning, there are several key differences between the two approaches:
Deep learning models automatically extract features from raw data, whereas machine learning models require manual feature extraction.
Deep learning algorithms scale with the size of the dataset, whereas traditional machine learning algorithms may plateau in performance as more data is added.
Deep learning models typically require more computing power and larger datasets to achieve high levels of accuracy.
When choosing between machine learning and deep learning, it is essential to consider the available resources, including computing power and labeled data. For smaller datasets and less powerful hardware, machine learning may be a more suitable choice.
Creating and Training Deep Learning Models
There are three common approaches to creating and training deep learning models:
Training from Scratch: This involves designing a custom neural network architecture and training the model using a large labeled dataset. This approach can be time-consuming and computationally intensive but may be well-suited for new applications or those with a large number of output categories.
Transfer Learning: Transfer learning involves fine-tuning a pre-trained model, such as AlexNet or GoogLeNet, to perform a new task. This approach requires less data and computation time, making it a popular choice for many deep-learning applications.
Feature Extraction: In some cases, deep learning models can be used as feature extractors, with the extracted features serving as input for a separate machine learning algorithm, such as a support vector machine (SVM).

Accelerating Deep Learning Models with GPUs Training deep learning models can be a time-consuming process, but the use of GPUs can significantly speed up the process. By harnessing the parallel processing capabilities of GPUs, training times for image classification problems can be reduced from days to hours.
The Future of Deep Learning and AI With the advancements in deep learning, AI is becoming an increasingly integral part of our everyday lives, driving the development of technologies such as driverless cars, personalized healthcare, and advanced automation. As deep learning continues to evolve and improve, we will likely see reach new heights, potentially even achieving the long-sought-after goal of General AI.
In conclusion, deep learning has revolutionized the field of artificial intelligence, enabling the development of powerful and versatile technologies across various industries. As computing power and data availability continue to grow, deep learning is poised to drive further advancements in AI, shaping the future of technology and the world we live in.
I hope you liked this article and learned something new. If you want to see more of my work, here are some links you can follow. On my website, you can discover my current and past projects and get in touch with me. On my Publishox blog, you can read my thoughts on various tech and innovation topics. On my Medium page, you can access my articles on different publications and platforms. You can also help me out by using this affiliate link, to products and services that I use and trust. Thanks for your support and have an awesome day!
Comments